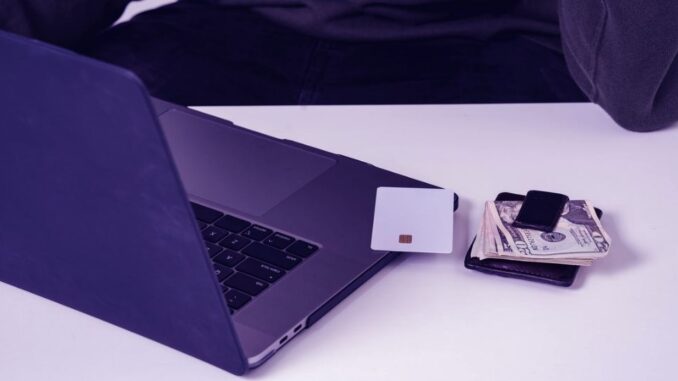
Digital Transformation in the Financial Sector as a Foundation for Automation
The digitalization of the banking sector continues to progress steadily. Data-driven methods such as process mining finance are becoming increasingly relevant. These approaches are not only used to visualize process flows but also to strategically identify automation potential. Particularly in highly regulated industries like banking, the efficient design of internal processes is essential for scalability, compliance, and customer satisfaction.
Banks handle a wide range of daily transactions, inquiries, and control processes. The use of Robotic Process Automation (RPA), workflow systems, and AI-driven services can lead to significant efficiency gains. However, the prerequisite for these improvements is a clear understanding of current operational workflows. This is where process mining finance comes into play: by systematically analyzing actual transaction and process data, it reveals manual steps, loops, or media discontinuities. This creates a data-based foundation for any form of automation strategy.
Beyond process visualization, process mining finance also enables continuous process monitoring. Deviations, delays, or unnecessary escalations can be automatically detected and evaluated. This makes it possible not only to optimize existing processes but also to plan new automated workflows in a targeted manner. Banks thus gain a reliable data basis for making investment decisions within the framework of their digital transformation.
Banking Processes with High Automation Potential
Not all processes within a bank are equally suitable for automation. Analysis using process mining finance reveals which processes are particularly standardized, rule-based, and high-volume. These criteria are ideal for successful implementation of automation solutions.
Processes with particularly high automation potential include:
- Loan application processing (e.g., data validation, scoring, document upload)
- Payment transactions (e.g., SEPA handling, error checking)
- Customer service workflows (e.g., address updates, status inquiries)
- Compliance-related queries (e.g., KYC checks, transaction monitoring)
- New customer onboarding (e.g., account opening, identity verification)
These processes are characterized by high repetition rates, clear rules, and defined interfaces. They offer an ideal environment for the integration of RPA tools or workflow engines. Structured process analysis through process mining finance highlights exactly where media disruptions occur, where manual intervention is needed, or where process loops develop.
Overview of Potential Processes
Process Area | Automation Level | Typical Tools |
Loan Application | High | RPA, Decision Engines |
Payment Transactions | Medium to High | APIs, Workflow Systems |
Customer Data Maintenance | High | CRM Integration |
KYC Checks | Medium | OCR, Rule-Based Engines |
Contract Archiving | High | DMS, Auto-Indexing Tools |
This classification is based on industry experience and empirical analysis within the banking sector. It shows how automation potential is distributed and where process mining finance offers particularly valuable insights.
Process Mining Finance as an Enabler for Data-Driven Automation Strategies
Process mining finance not only provides transparency regarding current workflows but also delivers quantitative decision-making support for strategic process changes. This includes throughput times, workload assessments, and deviation rates. These metrics can be used to prioritize and manage automation initiatives in a structured way.
A key advantage of process mining finance lies in its ability to identify so-called “happy paths” and “deviation paths.” This helps clarify how often processes actually follow the intended course—and where systematic issues arise. In combination with KPIs like “first-time-right,” escalation rates, or touchless processing rates, a clear picture of automation potential emerges.
In addition, process mining finance can be directly linked to performance dashboards to monitor ongoing automation efforts. For instance, it becomes clear whether a newly implemented RPA module actually accelerates processing or merely shifts tasks. This real-time monitoring is particularly valuable in regulated environments where documentation and traceability are required.
Strategically integrating automation and process mining finance enables organizations to make digitalization initiatives measurable. Decision-makers can use this data to invest in systems, training, or interfaces based on facts—and to monitor the value created by digital tools.
With this semantic breadth, process mining finance is positioned not just as an analysis tool but as an essential component of modern automation strategies. It lays the groundwork for digital efficiency, transparency, and scalability across the banking industry.
Leave a Reply